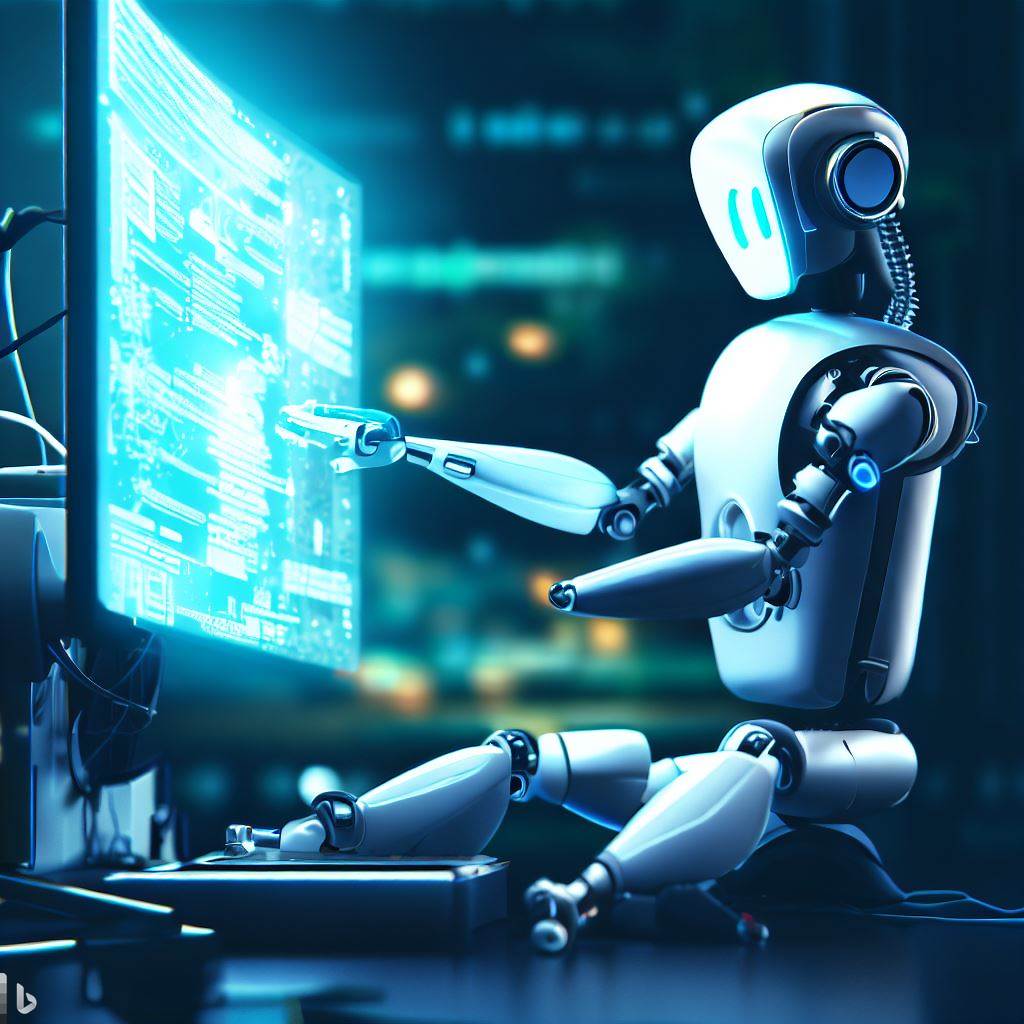
Yes, Large Language Models can Self-Improve.
A paper titled “LARGE LANGUAGE MODELS CAN SELF-IMPROVE “. proposes a method called Language Model Self-Improvement (LMSI) that enables large language models (LLMs) to improve their performance on reasoning tasks without using any ground truth labels. The LMSI method generates self-training data by using the LLM to answer questions and then uses those answers as training labels. The LMSI method also uses a multi-path decoding technique to generate diverse reasoning paths, which are used to train the LLM with self-consistency.
The results show that the proposed LMSI method can significantly improve the LLM’s performance on six different reasoning benchmarks, achieving new state-of-the-art results on three of them. The paper also demonstrates that the LMSI method can be used to distill knowledge from a large LLM to smaller ones. The authors plan to combine the LMSI-generated data with supervised data in the future to further improve LLM performance.
In plain English
The breakthrough in this research is that a type of artificial intelligence called a Large Language Model (LLM) can learn to improve its performance on a variety of tasks by generating its own training data without the need for external sources of labeled data or manual fine-tuning by humans.
In other words, the model can teach itself to get better and better at a task without needing humans to label large amounts of data or adjust the model’s parameters by hand. This is important because it can make it much easier and more efficient to develop and improve AI models, and it could lead to significant advances in a wide range of applications, from language translation to image recognition to medical diagnosis.
Here is the full paper: 2210.11610.pdf (arxiv.org)
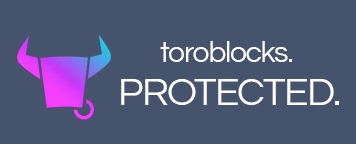